Welcome
Research in the Jin’s Research Laboratory is aimed at developing models for analyzing, assessing the performance and health conditions of engineering assets in smart manufacturing systems and complex engineering systems, and providing predictive and adaptive decision support tools for optimal operations, maintenance and control. Focus areas include:
– Predictive analytics for advanced manufacturing processes and systems
– Machine diagnostics and prognostics
– Sensor data analytics
– Physics-informed machine learning
– Roll-to-roll process for flexible electronics printing
– High-precision manufacturing processes
News
- Prof. Jin is awarded the 2020 Constantinos Mavroidis Translational Research Award by the College of Engineering.
- Prof. Jin received a $500k NSF Faculty Early Career Development (CAREER) Award for developing a Unified Sensing, Machine Perception and Control Framework for High-precision Micromanufacturing. (March, 2020) COE news
- Congratulations to Mengkai Xu on successfully defending his dissertation!
- Prof. Jin is awarded FY21 TIER 1 mentored project on Industrial AI-Assisted Synthesis of 2D Quantum Materials, with Prof. Swastik Kar.
- A new paper published: “A cross-disciplinary comparison of multimodal data fusion approaches and applications: Accelerating learning through trans-disciplinary information sharing”, in Expert Systems with Application, 2021, 113885.
- Prof. Jin is awarded a $2M NSF grant to develop Integrative Manufacturing and Production Engineering Curricula That Leverage Data Science in collaboration with colleagues Professors Kamarthi (PI), Issacs, Moghaddam and Jona.
- Congratulations! Ph.D student Anqi He received the 2019 John and Katharine Cipolla MS/PhD Award.
- Congratulations to Ph.D student Mengkai Xu’s paper on “Resiliency of Mutualistic Supplier-Manufacturer Networks” published in Scientific Reports [link]
- Dr. Jin was invited to present her roll-to-roll manufacturing research at the MIE department seminar in UMasss Amherest. [link]
- Dr. Xiaoning Jin (PI) is leading a $550K NSF grant on Precision Alignment of Roll-to-Roll Printing of Flexible Paper Electronics, in collaboration with Hongli Zhu (Co-PI) and UMass Amherst.
- Congratulations to PhD student Anqi He for winning the ASME Manufacturing Science and Engineering Conference Best Paper Award (1st Place), Erie, PA 2019. “Failure Detection and Remaining Life Estimation for Ion Mill Etching Process Through Deep-Learning Based Multimodal Data Fusion”
- Dr. Jin’s paper on “Virtual sensing and virtual metrology for spatial error monitoring of roll-to-roll manufacturing systems” is published in CIRP Annals [link]
- Congratulations to PhD student Xiaomeng Peng for receiving the 2018 John and Katharine Cipolla PhD Award!
- Dr. Jin is co-organizing a technical symposium on Advances in Data Analytics and Engineering Modeling for Intelligent & Resilient Manufacturing Systems at 2019 ASME International Manufacturing Science and Engineering Conference (MSEC), June 10-14, 2019, Pennsylvania State University. Papers are welcome to submit at https://www.asmeconferences.org/MSEC2019/login.cfm
- The Multimodal Data Fusion Workshop (MMDF 2018) Report is available now! [See the news][Archives]
- Xiaoning “Sarah” Jin is the guest editor of the Special Issue of IEEE Sensors Letters. [See details]
- Anqi He received CASE2018 Student Travel Grant to present his paper in Munich, Germany 2018
- Mengkai Xu’s new article on “A failure-dependency modeling and state discretization approach for
condition-based maintenance optimization of multi-component systems” has been published in Journal of Manufacturing Systems. [pdf] - Anqi He’s first paper on “NARNET-based Prognostics Modeling for Deteriorating Systems under Dynamic Operating Conditions” has been accepted by IEEE Conference on Automation Science and Engineering. Congrats!
- Dr. Jin is awarded a $750k project from Digital Manufacturing Design Innovation Institute (DMDII) on Predictive Factory research.
Research Highlights
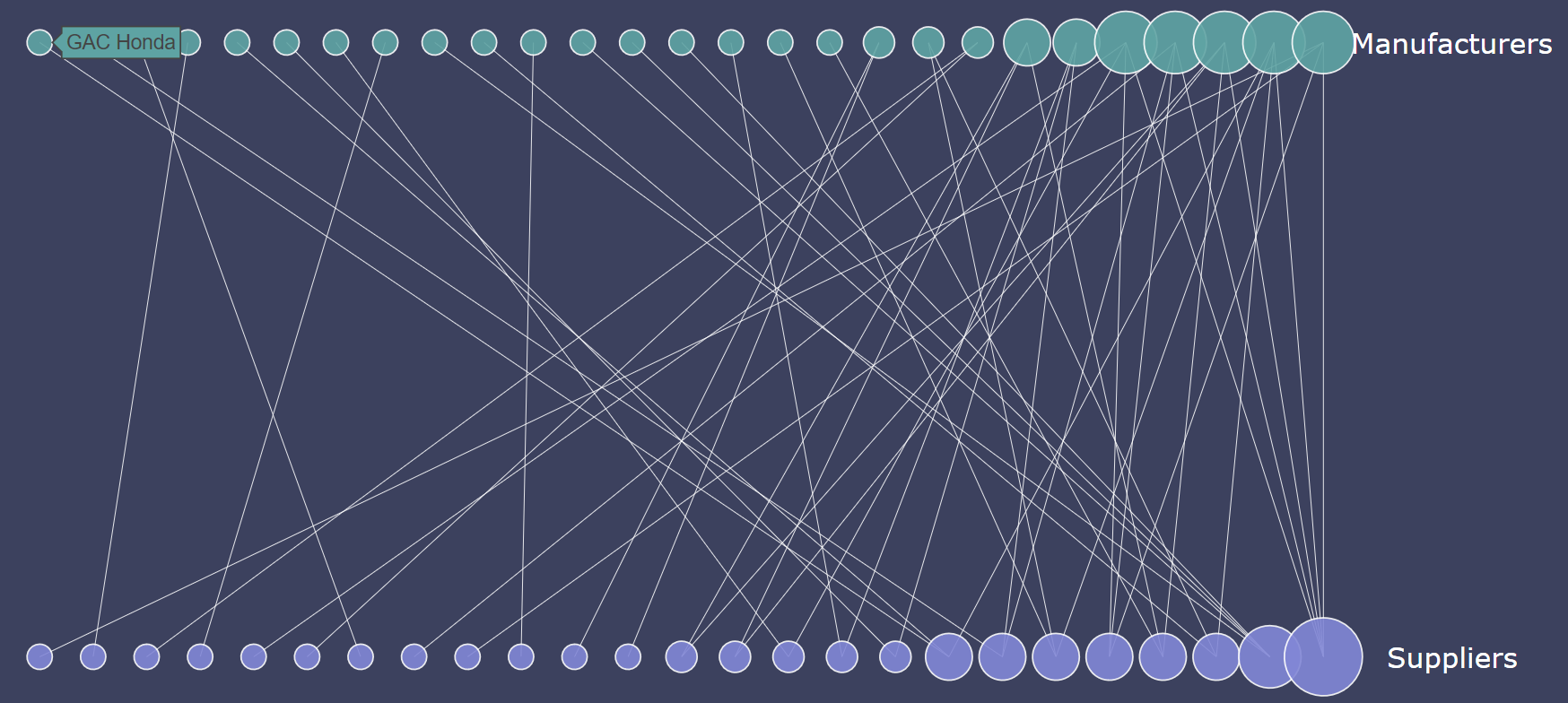
Resiliency of Mutualistic Supplier-Manufacturer Networks
Mengkai Xu, Srinivasan Radhakrishnan, Sagar Kamarthi, and Xiaoning Jin*
Current Supplier-Manufacturer (SM) networks are highly complex and susceptible to local and global disruptions, due to connectivity and interdependency among suppliers and manufacturers. Resiliency of supply chains is critical for organizations to remain operational in the face of disruptive events. In this work we investigate resiliency of SM networks using the quantitative methods employed to study mutualistic ecological systems. We create a bipartite representation and generate a multidimensional nonlinear model that captures the dynamics of a SM network and predicts the point of collapse. We extensively validate the model using real-world global automotive SM networks. The current work offers a means for designing resilient supply chains that can remain robust to local and global perturbations. An interactive visualization tool of the SM network and its resilience analysis has been developed by Capstone Project team. See https://rainbowfalcons.herokuapp.com/.
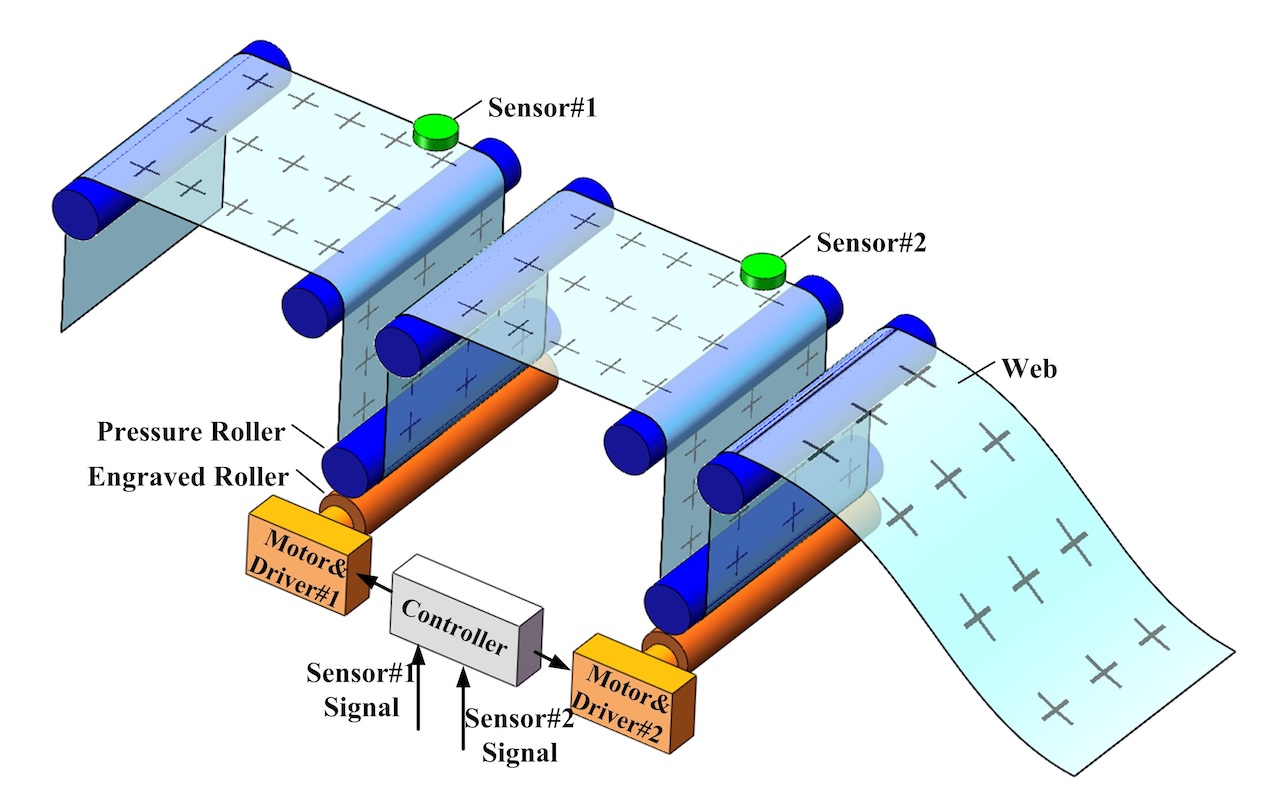
Roll-to-Roll Process Monitoring and Diagnostics
Huanyi Shui, Xiaoning Jin, Jun Ni
Roll-to-Roll (R2R) manufacturing has emerged as one of the most cost-effective manufacturing techniques for high-volume production of flexible printing electronics, thin-film batteries and solar cells, as opposed to low-volume batch production. However, in practice, real-time monitoring of the R2R process and in-situ measurement of fabricated materials during production are challenging because of the high speed and dynamic variation of the web. This paper aims to develop analytical models that can serve as virtual sensing and metrology tools to quantify process state variation and estimate product quality in R2R processes with limited accessibility of in-situ sensor.
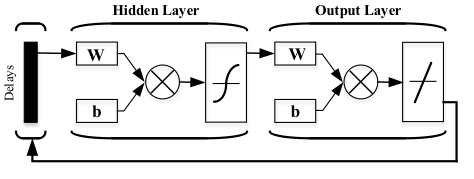
NARNET-Based Prognostic Modeling
Anqi He, Xiaoning Jin
This paper presents a new prognostics modeling method based on a nonlinear autoregressive neural network (NARNET) for computing the remaining useful life (RUL) of a deteriorating system under dynamic operating conditions. We particularly investigate how the degradation process is affected by the unit-specific operating conditions. The operating conditions are forecasted by a NARNET model based on the unit’s historical operating conditions. We show that the prognostics model integrating the operating condition forecast provides more accurate and efficient RUL prediction.
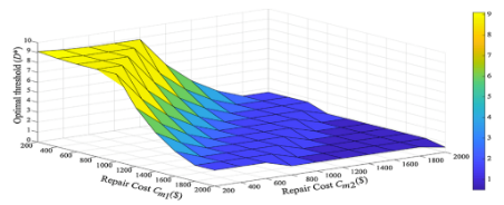
Stochastically-dependent Multi-component Degradation and Failure
Mengkai Xu, Xiaoning Jin, Sagar Kamarthi, Md. Noor-E-Alam
Unexpected component failures in a mechanical system always cause loss of performance and functionality of the entire system. Condition based maintenance decisions for a multi-component mechanical system are challenging because the interdependence of individual components’ degradation is not fully understood and lack of physical models. An extended proportional hazard model (PHM) is developed to characterize the failure dependence and estimate the influence of degradation state of one component on the hazard rate of another. An optimization model is developed to determine the optimal hazard-based threshold for a two-component repairable system.
Graduate Students
No funded graduate student positions are available at the moment, although applications are always welcome.
Post-docs
One post-doctoral position is currently available, please contact Prof. Jin for details.
Visiting Students/Scholars
Interested students/scholars from other institutions are welcome to contact Prof. Jin by email for the position of visiting students.